How to Collect a Keyword List for a Website
To successfully promote any type of website and get it to the top of organic search results, you always need to conduct proper keyword research and compile a comprehensive list of keywords, thematically relevant to your website's line of business, niche, and topic.
Not only is it useful for creating and optimizing a website, but also for launching advertising campaigns. Also, that's the ultimate way to get your website to the top of search results.
How to Collect Keywords for Your Website
You can do it manually and automatically. The latter includes specialized keyword research services. We will talk about them later.
During keyword research, keywords are selected and collected based on the analysis of the goods and services of your website, as well as the competitors' keywords. Also, the main attention is paid to keywords' statistics: search volume, seasonality, etc.
The underlying purpose is to create website pages for all search requests that meet the target audience's needs.
The process always includes:
- Collecting keywords that describe the website content in detail, taking into account its subject and purpose;
- Clustering collected keywords by meaning into sections and subsections.
- Optimization of all website pages for groups of collected keywords.
During keyword research for any e-commerce website, search requests must be divided into commercial and non-commercial queries.
Commercial queries include all keywords searchers use with an intent to purchase. They often tend to include words like "buy", "purchase", "price", "cost",etc.
Non-commercial queries are used by searchers to get certain information. They don't always lead the target audience to the website. However, there may be potential customers among such visitors.
Another important criterion is the keyword search volume.
A high search volume means that a certain keyword is searched for most often. Such keywords tend to accurately describe the industry, and they are used by your main competitors most often. Consequently, ranking for them at the top of search results is the hardest. Keywords with low search volume are used less often than others. Most often, they are long-tail keywords, consisting of several words. Their main advantage is lower competition for ranking at the top of SERPs. Also, they tend to be very specific and attract a warm target audience that knows exactly what they need.
How to Find Semantic Keywords
There are such ways to collect a keyword list online:
1. Collect the semantics in search engine services.
2. Use the semantic keywords of a competitor.
3. Collect keyword intersections of several competitors.
4. Apply keywords that competitors use in contextual advertising.
5. Collect similar keywords.
6. Use search suggestions.
The main semantic keyword tools are:
1. Google Keyword Planner
2. Serpstat
3. KWFinder
4. Keyword Tool
5. Wordtracker
6. WordStream
How to Find Competitors' Keywords
For creating your keyword list, using competitors' keywords is a must. You can find them automatically by using Serpstat.
Go to Site analysis, enter your competitors' domain → Keywords report:
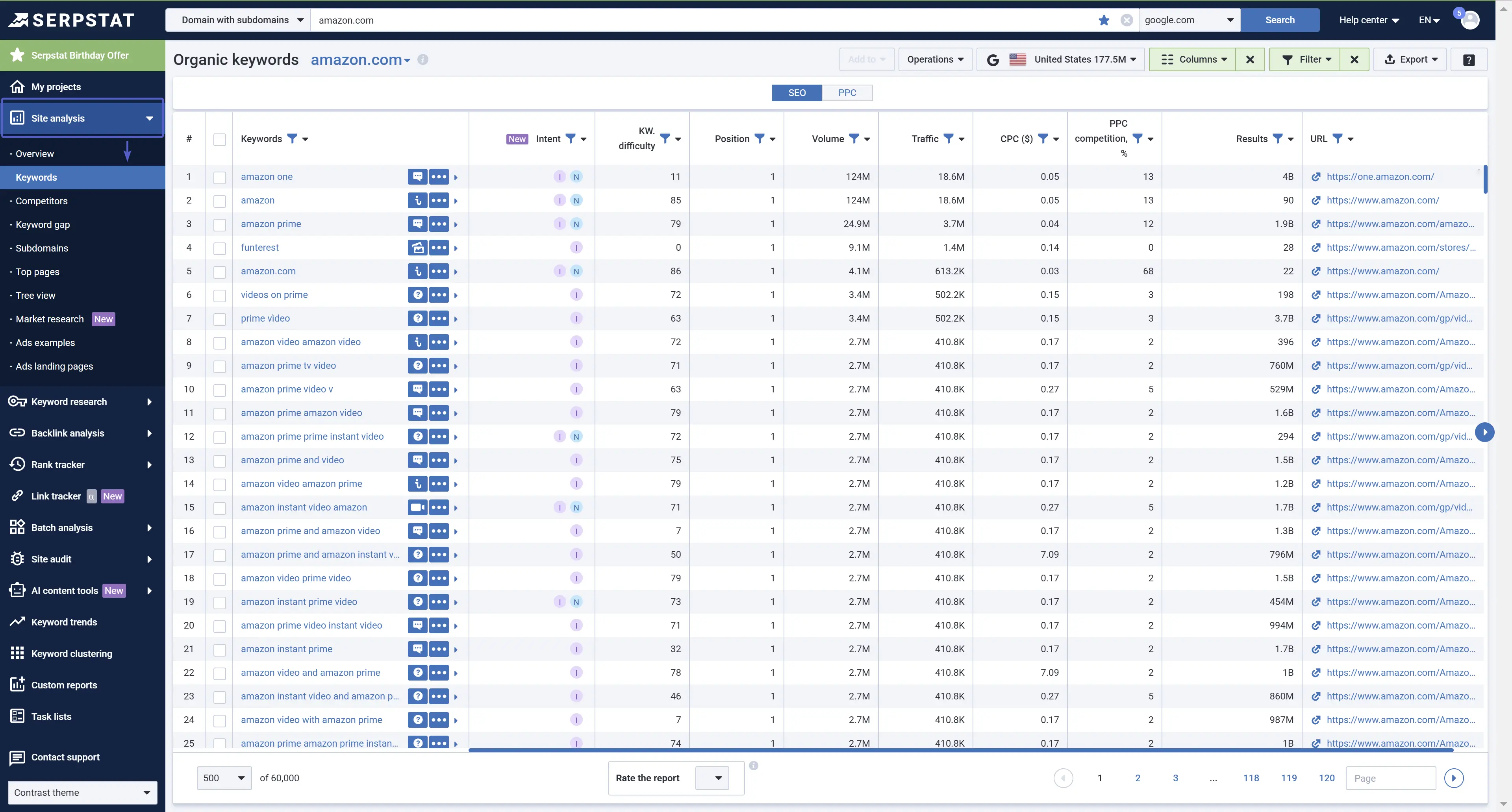
You can sort and filter the obtained list in the same report. Then, you can export the file in any convenient format.
Make sure to exclude all irrelevant queries, for instance, search requests with a competitor's brand name.
With the same method, you will be able to collect the keywords of several competitors.
Google Keyword Planner
In the service, select the "Find new keywords" option:
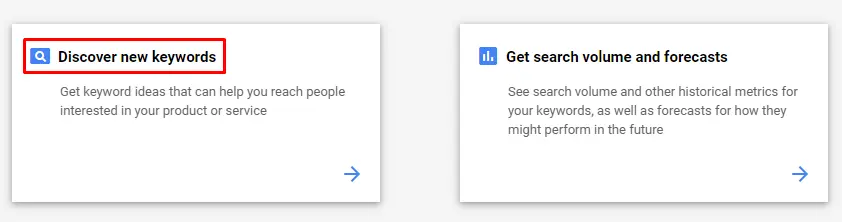
Specify the necessary website and click "Get Results":
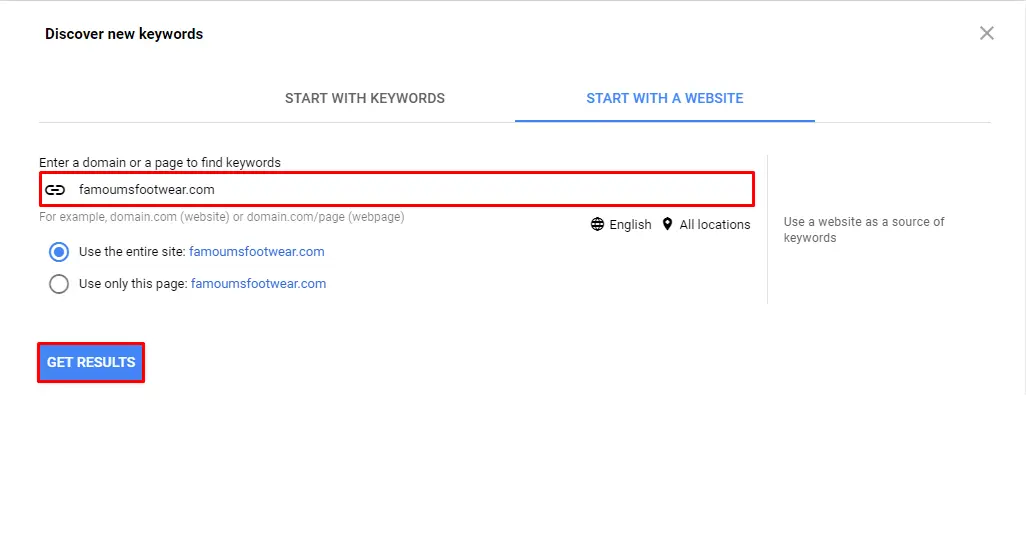
Now you have a list of keyword options with the average search volume per month. They are available for download:
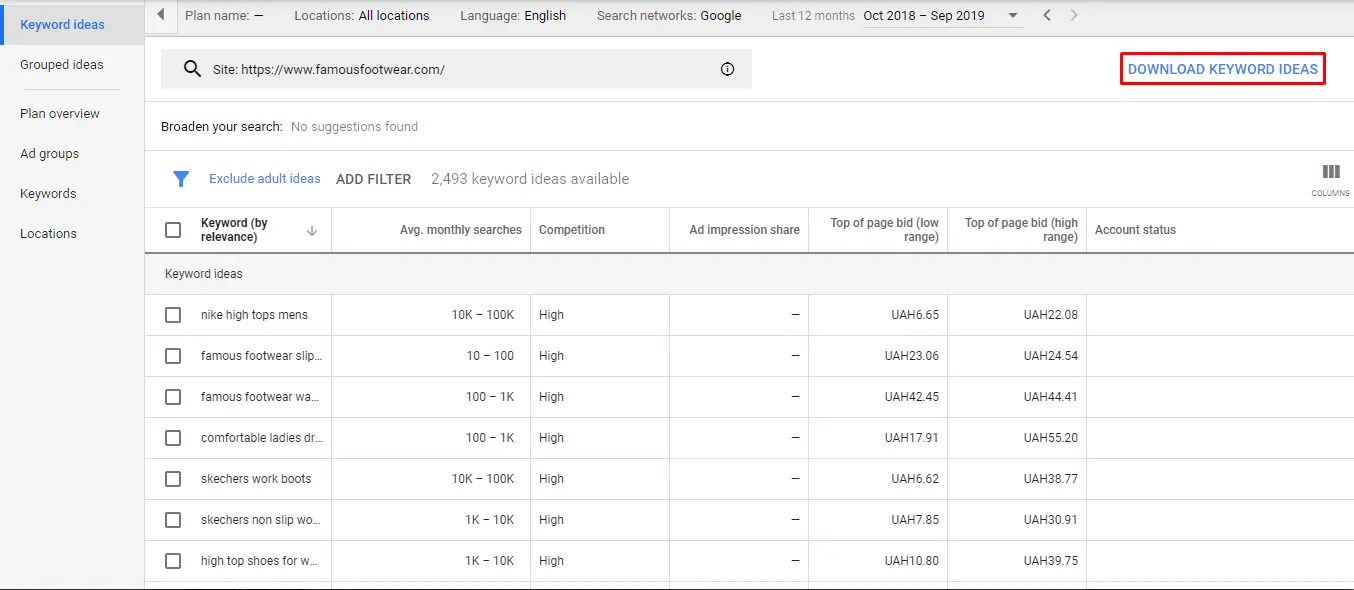
Keyword Tool
The service has a free and paid version. In the free version you can download a limited number of keywords:
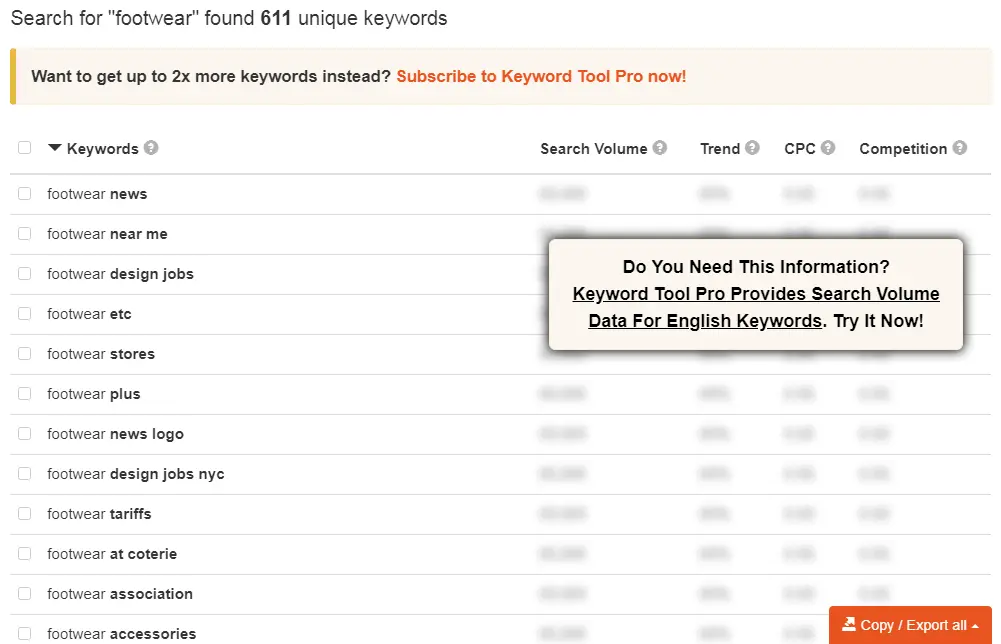
To get access to all data, you will have to purchase a subscription. However, you can also export the results and use them in Serpstat to get the advanced info.
Related Keywords and Search Suggestions
When users enter a search request into the search bar, search engines show them similar keywords and suggestions. These keywords should also be included in your keyword list. To collect similar search requests, you can use the following options:
Google
Similar keywords appear at the bottom of the page (SERP):
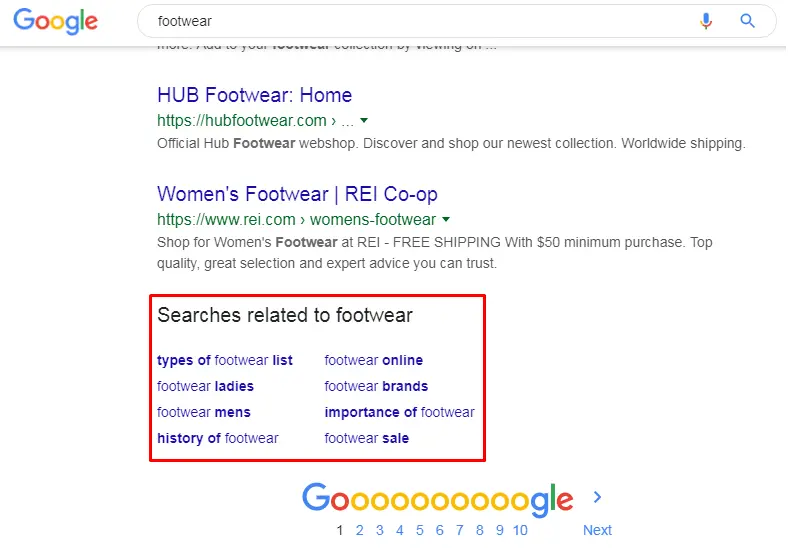
Search suggestions appear under the search bar:
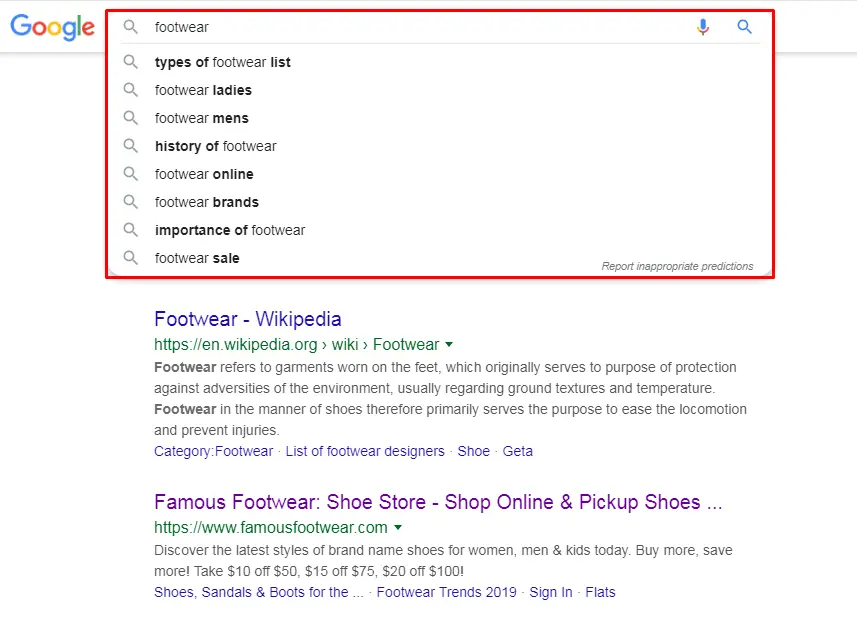
Serpstat
To find keywords with similar meanings, go to the Serpstat Keyword Research, enter your keyword, and select the search region → SEO Research → Related keywods.
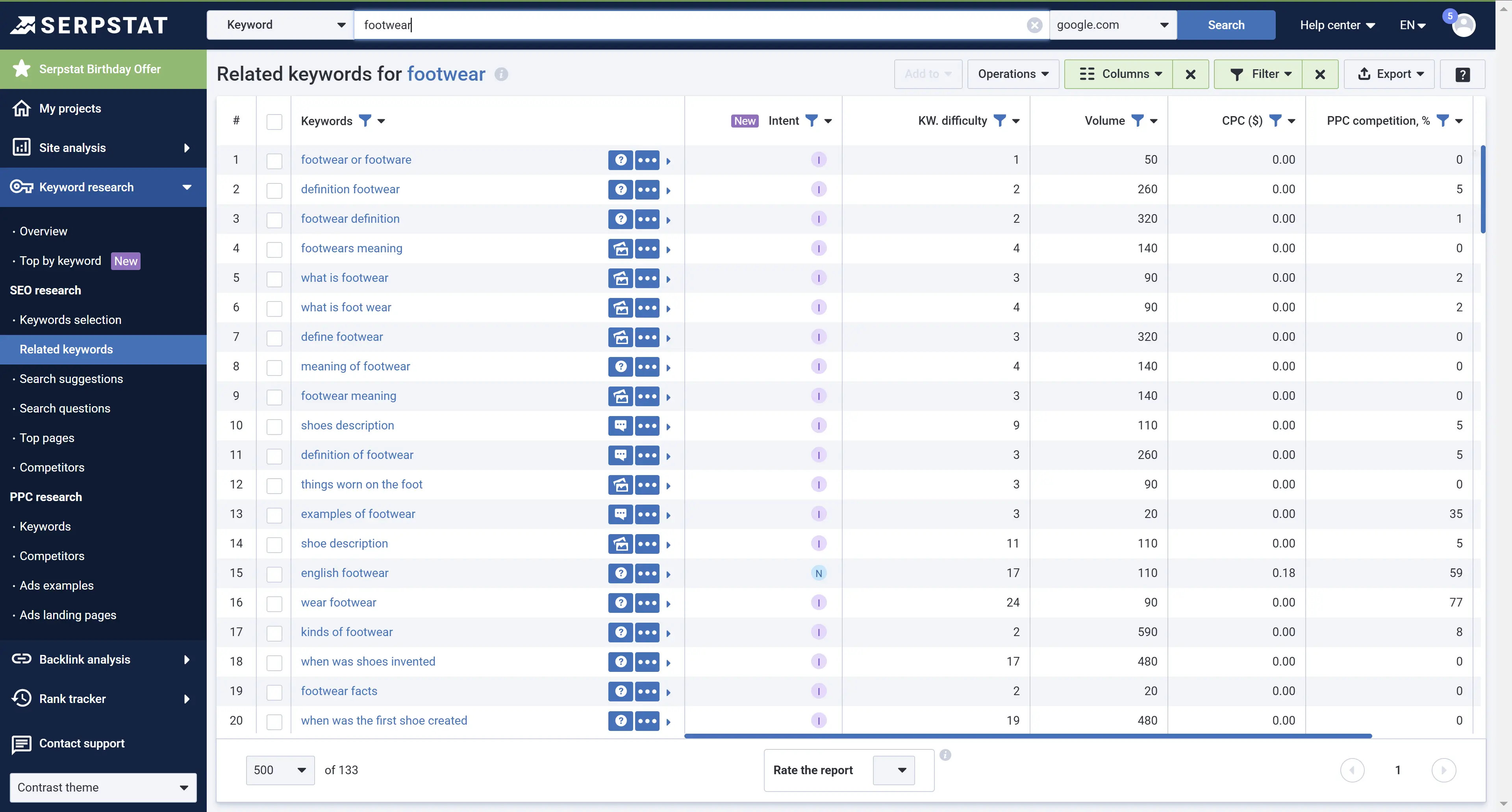
You will get the list of search suggestions in the corresponding report:
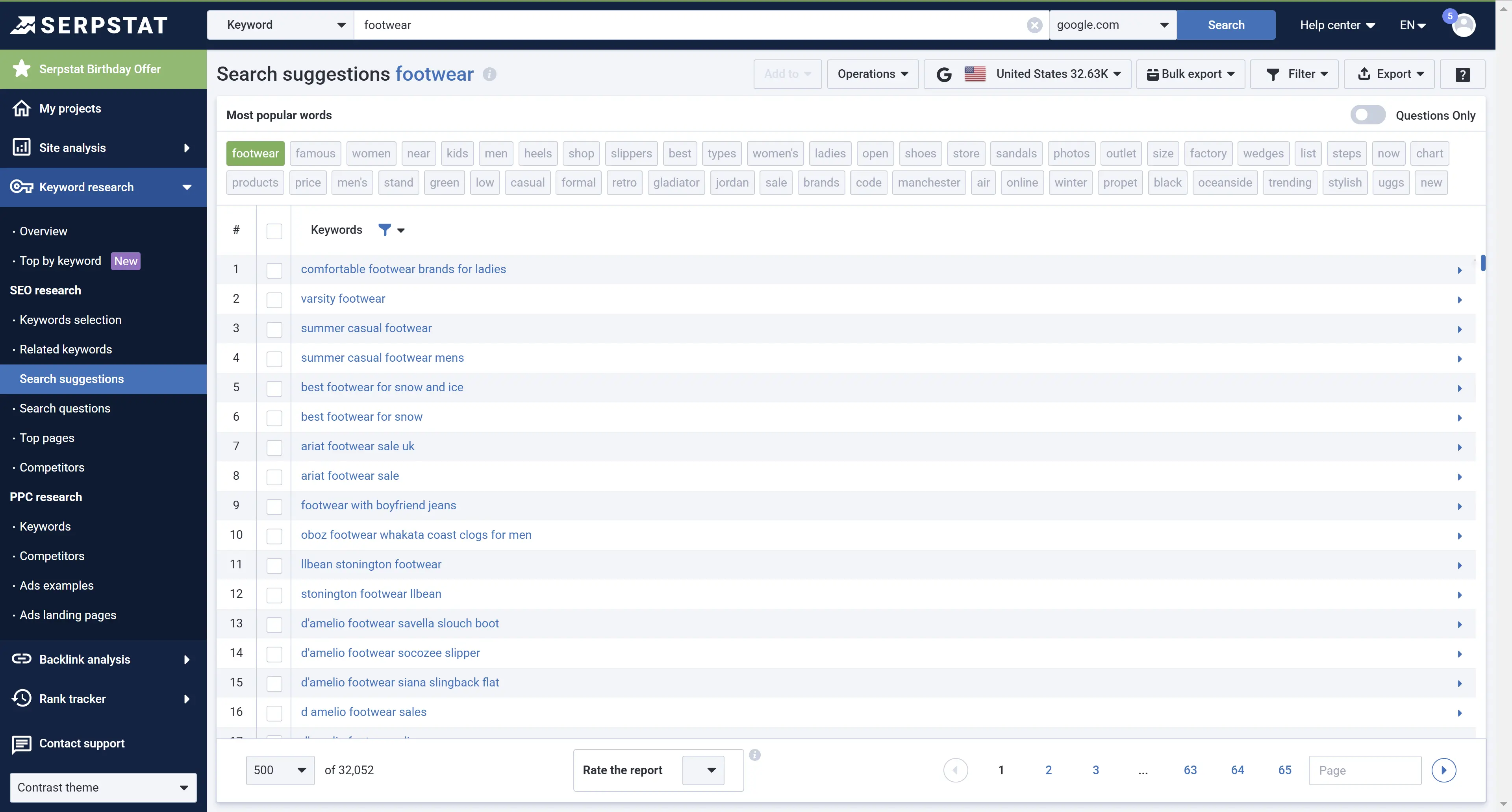
Compiling a Keyword List in KWFinder
To understand the principles of collecting keywords, let's take an online shoe store as an example. First of all, you need to think of search requests visitors can use to look for a similar online store. The most obvious request, potential customers would use is the word "shoes".
Run it through the KWFinder service to get information about it and other additional requests:
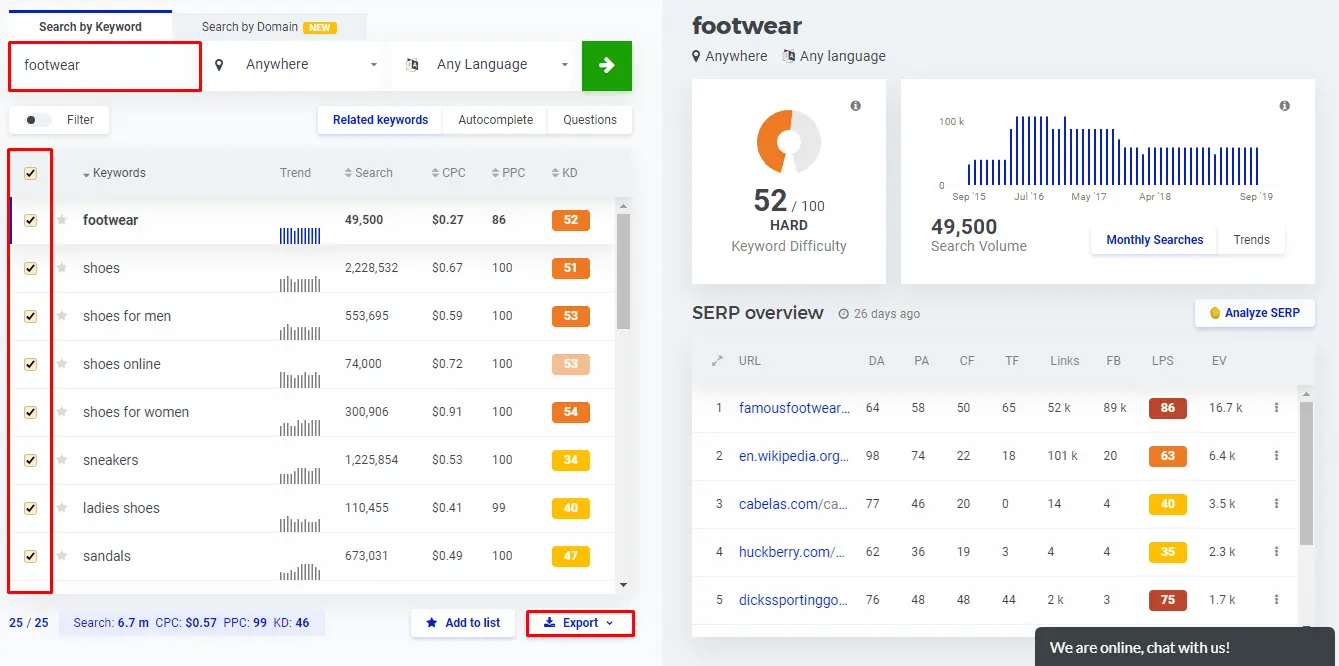
After adding the keywords, they can be exported or saved in the service to simplify further work.
To estimate how often users search for a given keyword, you can use the Search Volume metric:
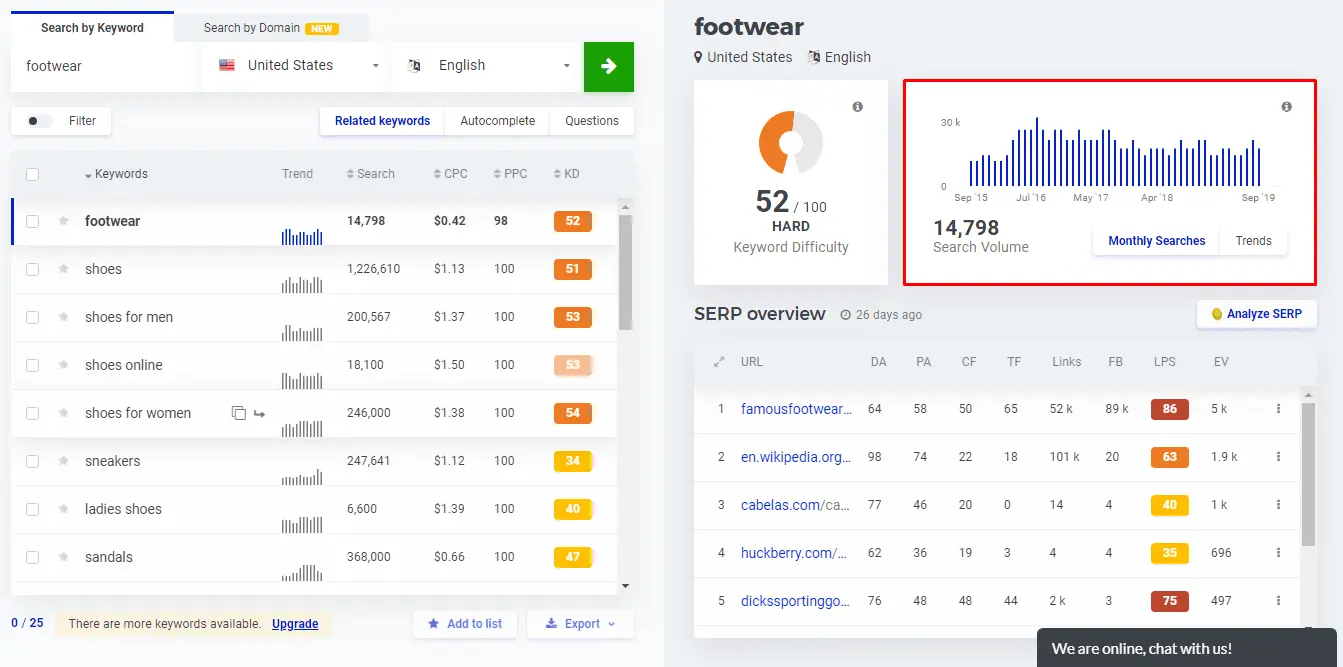
This data will help to find out the requests that are really the most popular, and find rarely used keys that have a high overall frequency. Thanks to this information, the site structure is being formed.
In this example, for promotion on the main page, high-frequency requests "shoes", "shoe website", "shoe store", "online shoe store" and "buy shoes" are suitable. The keys "baby shoes", "men's shoes", "women's shoes" and "summer shoes" are best suited for the sections. Low-frequency requests, for example, "sale of Italian shoes in London", are promoted on separate pages.
Similar work should be carried out with all types of shoes present in the online store, for example, to obtain statistics and select keys according to the words "boots", "sneakers", "shoes", "sandals", etc.
Collecting Keywords From Competitors' PPC Ads
Keywords competitors use for their contextual advertising are highly relevant, and you can add them to your keyword list. To find keywords from a competitor's PPC advertising campaign, use the Serpstat PPC Research report.
Go to Keyword Research → PPC Research → Keywords:
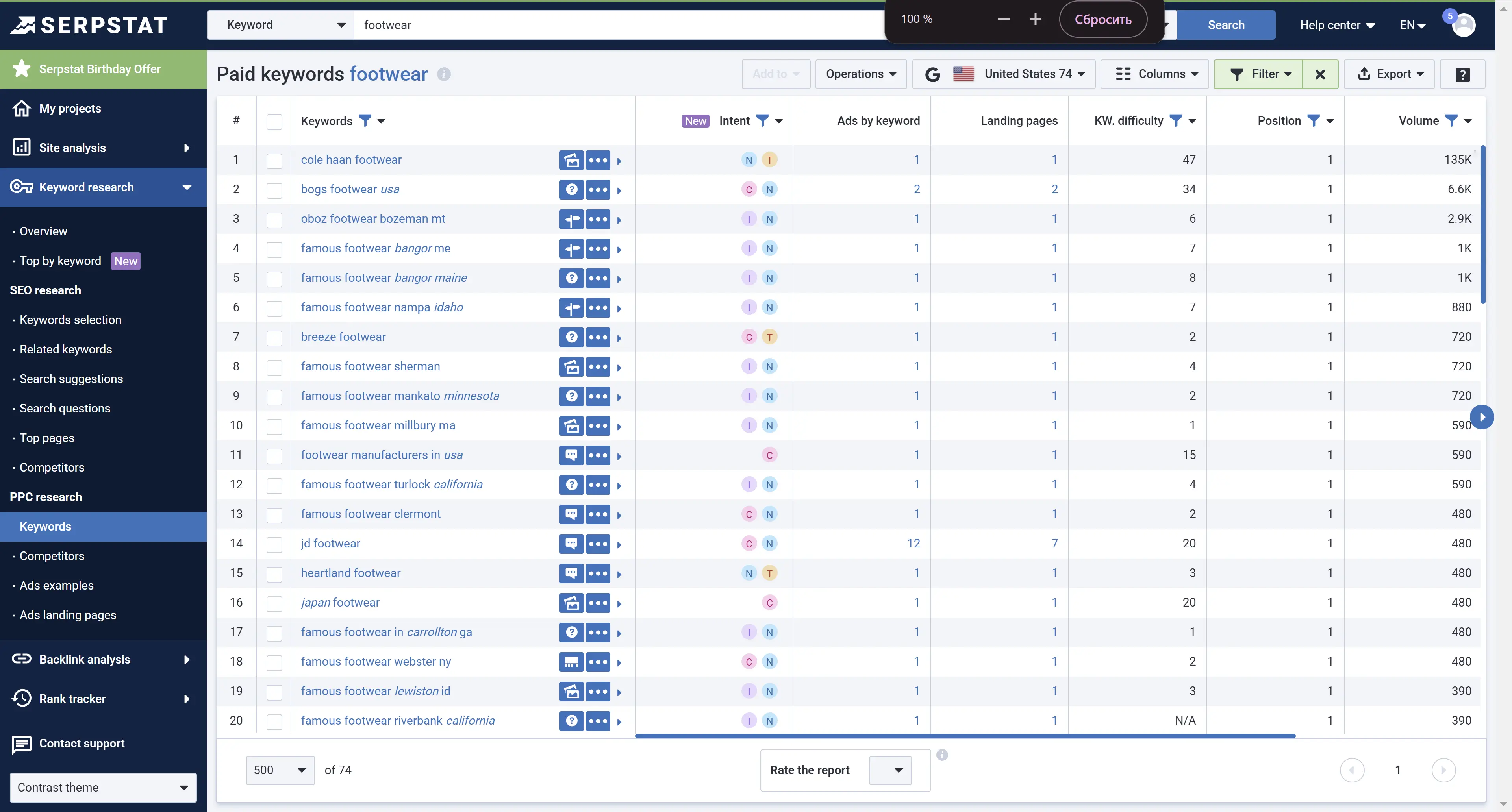
Each number is also clickable and will transfer you to the list of ads or landing pages for the given keyword.
Keyword Clustering
After the keyword list is assembled, proceed to the clustering. Clustering allows you to avoid mistakes associated with an incorrect combination of informational and commercial requests, which tends to have a detrimental impact on any website's rankings in search results.
For clustering select Keyword Clustering tool.
After importing keywords, they will be distributed into clusters:
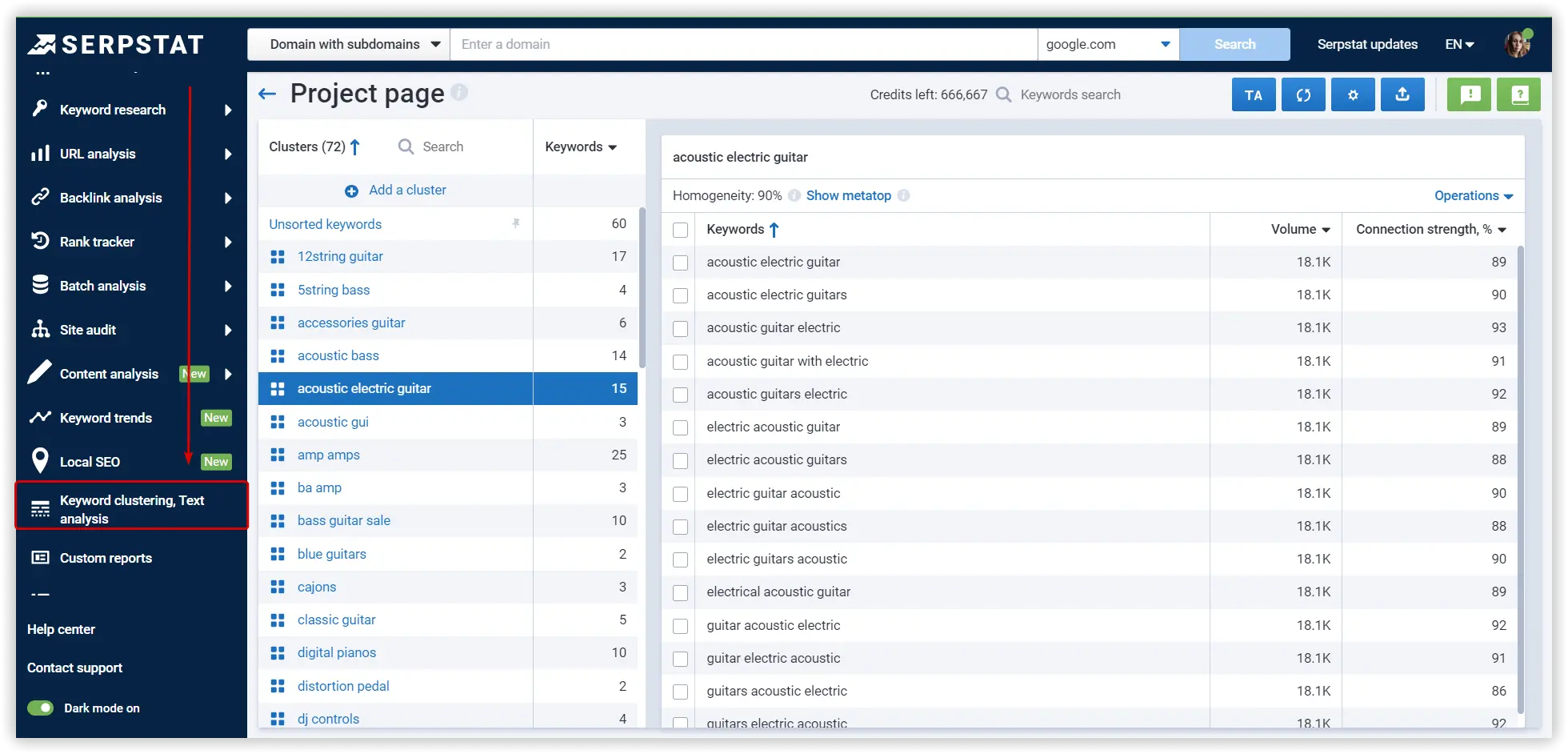
Start Finding
Keyword Ideas
Sign up and try for free
Discover More SEO Tools
Tools for Keywords
Keywords Research Tools – uncover untapped potential in your niche
Serpstat Features
SERP SEO Tool – the ultimate solution for website optimization
Keyword Difficulty Tool
Stay ahead of the competition and dominate your niche with our keywords difficulty tool
Check Page for SEO
On-page SEO checker – identify technical issues, optimize and drive more traffic to your website
Recommended posts
Cases, life hacks, researches, and useful articles
Don’t you have time to follow the news? No worries! Our editor will choose articles that will definitely help you with your work. Join our cozy community :)
By clicking the button, you agree to our privacy policy.